Data analytics security challenges, understanding and removing them!
Threats and vulnerabilities must be understood to prevent breaches in the complex data analytics security challenges environment.
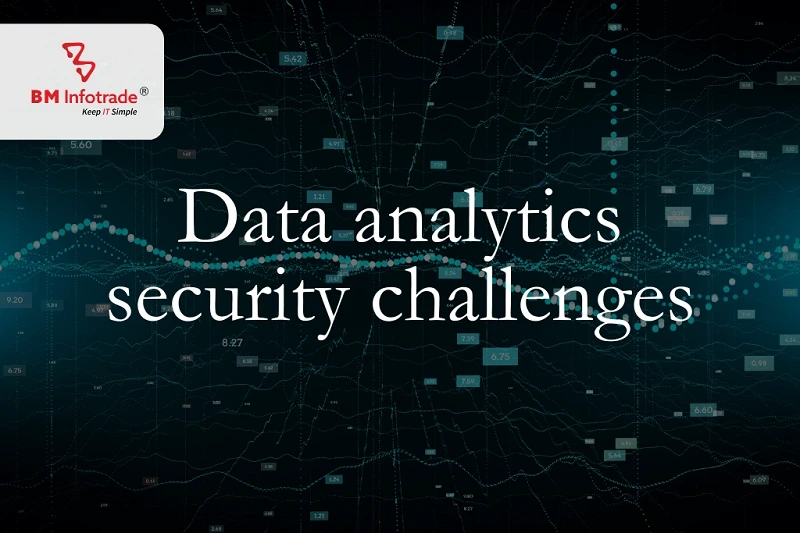
Data analytics security challenges, understanding and removing them!
Data analytics Security helps marketers, financiers, healthcare providers, and others make informed decisions in the digital era. Analytics helps firms gather insights, identify trends, and improve operations. The biggest challenge in this data-driven revolution is safeguarding large amounts of data in analytics pipelines.
This expanding data ecosystem is protected by cybersecurity. It covers all data security laws, methods, and processes to prevent hackers, unauthorised access, and other bad actors. In the complex data analytics network, cybersecurity protects important data availability, integrity, and confidentiality.
Data analytics and cybersecurity become more linked as companies utilise them to develop and compete. Emerging cybersecurity vulnerabilities need proactive management of the dynamic threat landscape.
This paper examines emerging data analytics security challenges, focusing on cybersecurity's role in privacy and data integrity. Through research and analysis, we aim to simplify data analytics security challenges by revealing existing cybersecurity challenges and offering solutions.
Table of Contents
Understanding Data Analytics Security Challenges
Threats and vulnerabilities must be understood to prevent breaches in the complex data analytics security challenges environment. Businesses employ data analytics to get insights and innovate, creating several cybersecurity risks. Data analytics security Challenges basics explain the complicated interplay between cybersecurity policies and the ever-changing area of data analytics.
Data Analytics Security Meaning
Data analytics security protects data availability, integrity, and confidentiality by merging cybersecurity with analytics frameworks. It involves access control, encryption, threat detection, and incident response to mitigate insider and external threats.
Analytics Data Security Value
The data analytics aphorism "data is the new oil" highlights data's strategic importance to organisational performance. As companies utilise data analytics to make decisions and get insights, data security becomes crucial. Data breaches can result in legal issues, regulatory noncompliance, financial loss, and reputational damage.
Key Data Analytics Security Elements
A good cybersecurity posture in data analytics security analysis relies on several key factors. Data security relies on encryption, which prevents unwanted access to private data. Access control solutions like MFA and RBAC restrict data flow in analytics environments to prevent unauthorised access. Continuous monitoring and threat detection can also help businesses notice and resolve cybersecurity issues rapidly.
Data analytics security challenges businesses face both benefits and drawbacks from emerging technologies like Machine Learning. Organisations may improve their cybersecurity by using Machine Learning algorithms to spot irregularities and potential threats in real-time. Machine Learning algorithms generate new attack vectors as attackers target AI-driven cybersecurity solutions.
New Data Analytics Security Risks
As companies embrace data analytics' revolutionary promise, new threats emerge. In this section, we discuss insider threats, data breaches, and AI and cloud computing vulnerabilities. In a changing environment, threat detection is essential for data analytics ecosystem security.
Data Breach
Data breaches threaten data analytics security and can harm people and organisations. These breaches can result from insider threats, accidental data disclosures, and foreign cyberattacks. Recent high-profile data breaches demonstrate the need to improve threat detection to swiftly detect and repair breaches.
Internal Threats
Even while external attackers dominate data security discussions, insider assaults pose a substantial threat to data analytics ecosystems. Insiders can intentionally or unintentionally compromise secret information and organisational integrity and confidentiality. Strong threat detection systems must detect odd activity and stop insider threats before they become security issues.
Machine Learning/AI Vulnerabilities
Due to the rapid growth of AI and Machine Learning, data analytics training alternatives have increased. When attackers exploit AI models and algorithm faults to bypass threat detection systems, these technologies introduce new vulnerabilities. AI-powered threat detection systems are defeated via model evasion, data poisoning, and adversarial attacks. This highlights the need for effective AI-related risk protections.
Cloud Security Risks
Cloud data analytics might be more versatile, scalable, and cost-effective, but also increases cybersecurity threats. Cloud-native analytics systems need powerful threat detection capabilities to prevent misconfigurations, unauthorised access, and data breaches. To reduce these risks and secure cloud data, restrictive access restrictions, and cloud-native threat detection are essential.
Regulatory Compliance Issues
Compliance with tight data collection, storage, and usage regulations complicates data analytics security challenges. Non-compliance violates customer trust and exposes firms to legal consequences. To comply with regulations, organisations must use effective threat detection methods to proactively identify and address cybersecurity concerns.
Read More: The usefulness of VAPT in Cyber Security, Tools, and Testing
Prevention Methods
As new threats emerge in data analytics security challenges, businesses must build proactive mitigation methods to prevent breaches and vulnerabilities. This component provides a comprehensive data analytics cybersecurity framework with staff training, access restriction, encryption, and regular monitoring. We also examine how threat detection can identify and mitigate data analytics privacy risks.
Anonymize and encrypt data
Data encryption prevents unauthorised access and interception. Data in transit and at rest can be encrypted to reduce data breaches and unauthorised disclosures. By masking and tokenizing data, firms may protect privacy while gaining insights from data analytics.
Access and authentication mechanisms
Effective access control is needed to protect sensitive data and prevent unauthorised people from compromising it. Strong identity management systems, multi-factor authentication (MFA), and role-based access control may mitigate insider risks and establish granular access restrictions. Strong authentication can improve cybersecurity and lessen privacy issues from unauthorised access.
Threat Detection and Monitoring Constantly
Continuous monitoring is needed to detect abnormalities and cybersecurity hazards in real time. Modern threat detection and analytics may instantly identify suspicious behaviour, unauthorised access attempts, and other security events. Businesses may also prevent data breaches and decrease privacy concerns by using AI and machine learning algorithms to detect threats.
Employee Training and Awareness
Technology security is important, but human mistake still makes data analytics ecosystems vulnerable. Staff members learn cybersecurity best practices, data handling rules, and privacy regulations through extensive training to identify and mitigate privacy dangers in their everyday work. By promoting security awareness, organisations may improve cybersecurity and decrease data breaches.
Read More: The Role of SOC Experts in Enhancing Organizational Security
In summary
To secure sensitive data and cybersecurity resilience, companies must navigate emerging data analytics security challenges with proactive measures and effective policies. In this last chapter, we discuss the most critical lessons from our data analytics cybersecurity research and the importance of anomaly detection in risk mitigation and data integrity maintenance.
This research explored emerging data analytics security challenges such as insider threats, cloud security, data breaches, and AI vulnerabilities. We've discussed how access restriction, encryption, and continual monitoring may reduce these threats and protect data privacy, availability, and integrity.
Anomaly detection is the core of data analytics cybersecurity, allowing firms to swiftly identify and eliminate threats and suspicious activities. Using advanced analytics and machine learning algorithms, organisations may recognise aberrant behaviours, unauthorised access attempts, and deviations from regular activity that may indicate a security or privacy breaches.
As firms adopt new technologies, deal with complex laws, and adapt to changing cybersecurity threats, data analytics security challenges will alter. AI, Machine Learning, and anomaly finding can improve cybersecurity resilience and enable proactive attack mitigation.
Given the shifting cybersecurity landscape, we urge firms to prioritise data analytics security challenges and invest in powerful anomaly detection technologies, proactive mitigation methods, and extensive staff training. Using cutting-edge cybersecurity technologies and promoting security awareness may help organisations defend against emerging attacks and safeguard analytics data.
Anshul Goyal
Group BDM at B M Infotrade | 11+ years Experience | Business Consultancy | Providing solutions in Cyber Security, Data Analytics, Cloud Computing, Digitization, Data and AI | IT Sales Leader