What Are Large Language Models (LLMs)? A Complete Guide to AI-Language Models
The face of technology is changing and so is our relationship with it as AI continues to shape our world. Leading this change are Large Language Models (LLM).
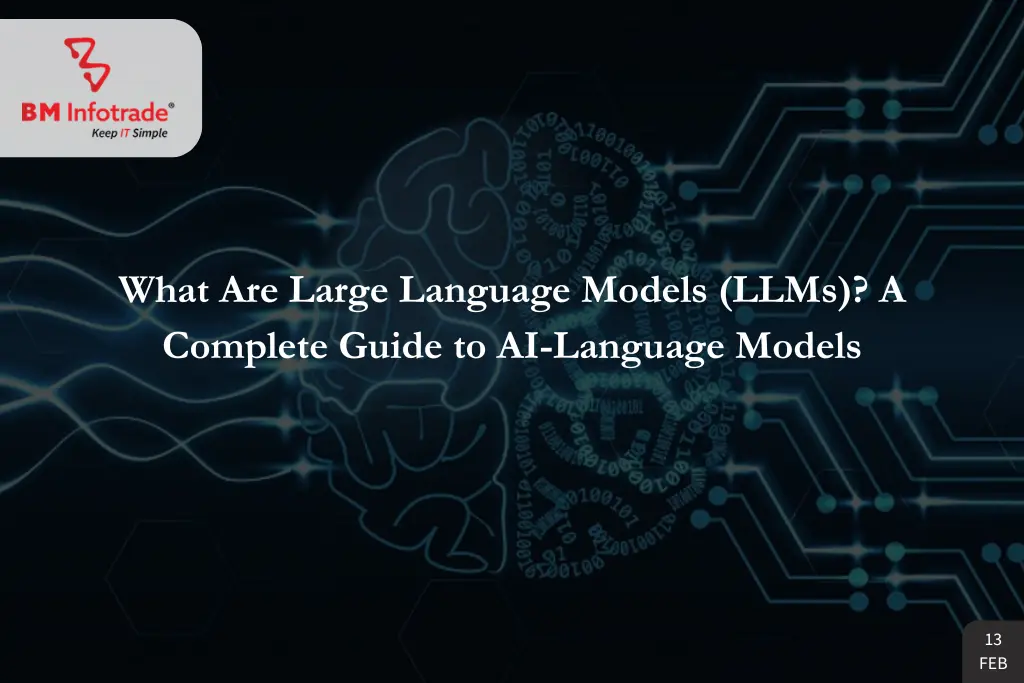
What Are Large Language Models (LLMs)? A Complete Guide to AI-Language Models
Table of Contents
The face of technology is changing and so is our relationship with it as AI continues to shape our world. Leading this change are Large Language Models (LLM). Such models are specifically created to generate and comprehend text as a person would. They can be, therefore, very useful in many areas including customer support, content creation, and data analytics. We will take a look at what LLMs are what LLMs are, how they function, where they can be used, and why their relevance continues to grow.
What Are Large Language Models (LLMs)?
Large language models (LLMs) are a specific type of advanced artificial intelligence (AI) system that employs deep learning architectures to process, create, and modify human language. LLMs are trained on large data sets that include texts from books, websites, and other sources, allowing them to analyze data and provide answers that make sense and are relevant to the context. BERT and GPT-4, for example, can engage in scripted dialogue, translate text into other languages, and complete other tasks involving content creation mimicking human writing patterns.
Large Language Models are high-level language-based statistics-based algorithms that have high definition in the volume of data and the number of parameters they have. These parameters are most times expressed as billions and trillions, implying the capability even LLM itself is capable of acquiring complex language structures making it almost impossible for such systems to provide erroneous responses.
How Do Large Language Models Work?
There is a technology called Transformer Architecture that is used in LLMs which is capable of processing and understanding sequences of data such as languages. This is a summary of how LLMs work in detail: Data Collection and Training: Large Language Models are used in the system and they are trained from large databases of text which have their origin in books, articles, websites and other forms of written texts. Therefore, they acquire knowledge concerning grammar, context, the relationship between the words and the general characteristics of different written texts.
- Contextual Understanding: One of the key unique features of LLMs is their potential to discern contexts. Most LLMs possess attention mechanisms which allow examining certain parts of the input text thus determining what is important. This step allows LLMs to produce correct and relevant breezes.
- Text Generation: Later on, LLMs can generate texts simply by looking into a certain context and predicting what the next word in the sentence should be. The outcomes of the model predict the next likely word from the ones available. This enhances the ability to elaborate long and precisely accented sentences that sound “natural” or “human” like.
Key Features of Large Language Models
We can list many reasons why LLMs stand out as powerful tools in AI. Copies of LLM Prompts have several features that make them stand out:
- Versatility: LLMs can perform a variety of tasks ranging from translating to writing or programming in different languages. This multi-tasking capability proves beneficial in a variety of sectors.
- Context Awareness: Exposed to so many different data, LLM is better at answering questions than standard AI models. It provides very appropriate and relevant answers many times even when the questions are complicated.
- Scalability: Here, we can multiply LLMs beyond our wildest dreams and put so many tasks at their fingertips. This makes it perfectly useful in customer support, where timing and accuracy can sometimes make or break a deal.
- Personalization: By training them in a certain way, LLMs can be adapted to meet specific industry or business requirements. They may, for example, get training in medical literature and be masters in the provision of such information in the healthcare field.
Read More: What Are DNS Zones and Records? A Complete Guide to Understanding DNS Basics
Applications of Large Language Models
LLMs are gaining importance in multiple industries. Let us consider a few of these in good detail:
- Customer Service: Now, LLMs are used to manage customer interaction through their virtual chatbots and assistants. Such models can provide quick and accurate answers, leading to improved service quality and overall satisfaction.
- Content Creation: Thinking of writing articles, social media posts and marketing copy? Well, Whose suggestions!? No, Seriously! LLMs Content Creation: LLMs doesn’t seem like a name, more like a forced suggestion on a Google search. Sounds like a lead generator doesn’t It?
- Social Language: As an example, Google Translate which includes LLM can be used to remove language barriers. Not many people use taxis. LLMs allow accurate translation that takes into account the meaning of the original and is used contextually.
- Intelligence Team: LLMs inspire dramatically increased productivity allowing the usage of complex software models for low-ve level coding. These models facilitate complex tasks making low-level coding a matter of lines. That eats less time.
- Model Output Research: Think of deep learning for teams where summary considerations and understanding are presented as key features for research and decision-making. What does this suggest? That it is required in medicine and healthcare which is very complex or vast for any language LLM.
LLM Disadvantages That Businesses Need to Overcome
Large Language Models seem to have brought more bad than good. For business development plans, this might as well be a resurrection without the good old-fashioned EVIL!
- Limiting Workers Repetitiveness: By needlessly outsourcing your IT needs, LLM seems to slap high-level human engagements like support and marketing efforts in a microwave. Which does more time wasting if not increasing time and resources.
- Indestructible Structure: Adapting LLM to various industries seems pretty straightforward; however, it is extremely inefficient for mass repetitive projects LLM is way more efficient.
- Cost-Effectiveness: To a large extent, the automation of LLM LSS eliminates the need for a human resource in the delivery of services like customer support and content generation. Businesses do not have to incur the extra cost of manpower by deploying LLMs to do the work.
- Improved User Experience: LLMs understand and respond to context so the user experience is more intuitive and better suited to the needs of customers. This is especially important in e-commerce and customer support services where the users’ contentment must always be upheld.
Challenges and Limitations of LLMs
Above and beyond these capabilities angel LLMs prove to be equally encumbered by challenges:
- Bias: If the data is used to train LLMs, hence the outputs will be biased as well. So the developers have to tread carefully and apply bias mitigation plans to strike some balance.
- Resource Intensity: The types of hardware and environmental resources used for the training phase of LLMs, as well as their operational phase are vast. This renders the horizontal and upkeep of such LLs cost-intensive as well as not sustainability-friendly.
- Lack of Real-time understanding: LLMs are capable of crafting text based on the past but don’t adapt well in a fast-moving environment unless they are retrained or fine-tuned.
- Security & Privacy Issues: privacy breaches are a potential risk and these concerns can be exacerbated by the sheer volume of data that LLMs have been trained on. It is critical to address and implement risk-based management to protect these models in the deployment phase.
The Future of Large Language Models
LLMs boast of a good future that will see progressive research and development in their advancement and limitations. Several trends and advancements can be traced.
- Increased Incorporation of Real-time Information: This is expected in the coming years and it will make LLMs more responsive since they will hash out the need for real-time data sources.
- Building Inclusive AI: While this goal may be quite elusive, developers are keen to ensure LLMs don’t generate biased outputs regardless of the use cases.
- Sustainability: While the versatility of LLMs is desirable, the LLMs of the future should be more eco-friendly.
- Increased Domain specificity: Shortly, LLMs would likely be domain-specific, hence better accuracy, as they would have versions that are more specialized to the healthcare, finance, or legal services industries.
Read More: Unlock Success: ROI-Driven Executive Strategies for Maximum Business Growth
Conclusion
As in the case of LLMs, it can be anticipated that there will be a transition in the development, deployment, and use of artificial intelligence and technology. The realm of customer service enhancement can be achieved using LLMs to make the creation of content or even coding automatically. Even though they have some negative attributes such as bias and high consumption of resources, newer developments may pave the way for the effective utilization of these models shortly.
Anshul Goyal
Group BDM at B M Infotrade | 11+ years Experience | Business Consultancy | Providing solutions in Cyber Security, Data Analytics, Cloud Computing, Digitization, Data and AI | IT Sales Leader