Data Science vs Data Analytics: Differences and Synergies
Data Science vs Data Analytics: Businesses rely on data for strategic decision-making and unique insights in the digital age.
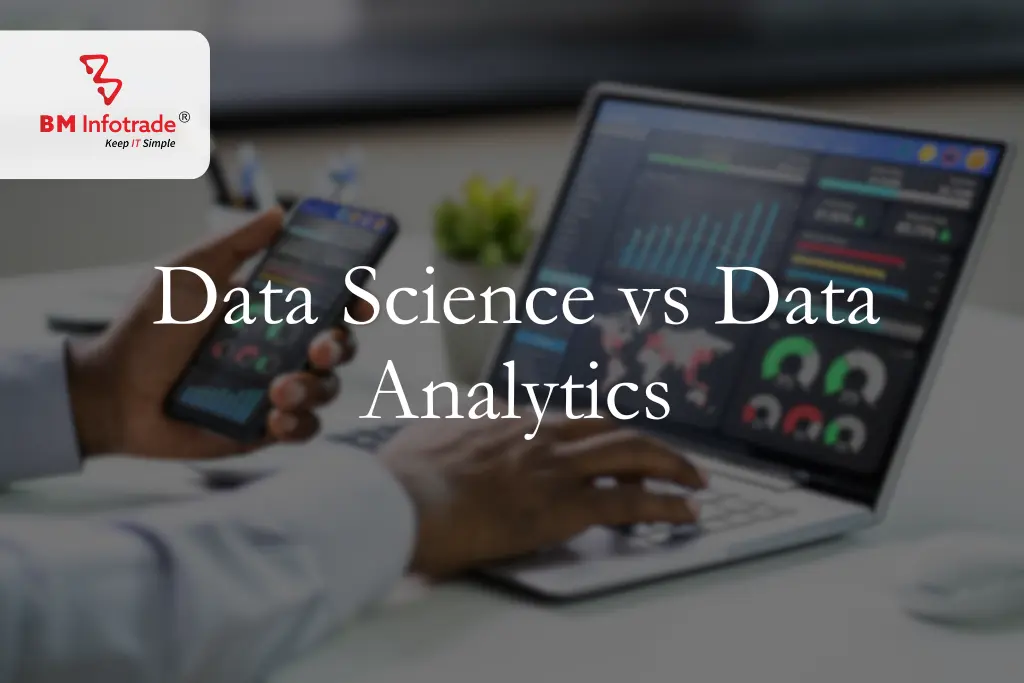
Data Science vs Data Analytics: Differences and Synergies
Data Science vs Data Analytics: Businesses rely on data for strategic decision-making and unique insights in the digital age. Data Science and Data Analytics are leading this data revolution. The phrases are often used interchangeably, but a closer study shows specific aspects and synergies that contribute to their aggregate influence.
The growing complexity and volume of everyday data have encouraged organisations to adopt data-driven strategies for competitiveness. Data-savvy workers are in demand as businesses realise they need to gain actionable insights from massive amounts of data.
Data Science vs Data Analytics are explained in this blog, including their differences, purposes, and methods. We want to help aspiring and experienced data professionals navigate the changing world by examining their differences and collaboration possibilities.
Before exploring, we must understand the Data Science vs Data Analytics context. Technology, data, and subject expertise have created revolutionary capacities beyond standard business methods.
Table of Contents
Understanding Data Science
Definition and Scope of Data Science
The goal of the broad area of data science is to extract knowledge and insights from complicated and unstructured data through various activities. To find patterns, provide forecasts, and promote well-informed decision-making, entails the integration of numerous methodologies, algorithms, and domain expertise.
Key Components of Data Science
Data Collection and Preprocessing:
Initially, data scientists collect unprocessed data from many sources. To guarantee data quality and consistency and set the stage for further analysis, this entails cleaning and preprocessing.
Exploratory Data Analysis (EDA):
EDA stands for exploratory data analysis, the process of looking through and analysing data to find trends, patterns, and connections. This stage assists in developing hypotheses and providing direction for further modelling decisions.
Machine Learning and Predictive Modelling:
Using machine learning algorithms to create predictive models is at the heart of data science. These models can be trained to predict or categorise previously unseen data using past data.
Feature Engineering and Selection:
To improve model performance, data scientists methodically create features from unprocessed data. By ensuring that only the most pertinent variables are included, feature selection helps to streamline the modelling process.
Model Deployment and Evaluation:
Careful model evaluation is necessary to determine the models' generalizability and correctness. After that, effective models are implemented in actual settings, enhancing data-driven decision support systems.
Read more:- Data and AI
Data Science Applications in the Real World
Applications of data science can be found in many different industries:
- Healthcare: Personalised treatment regimens and disease diagnoses by predictive modelling.
- Finance: Algorithmic trading, risk assessment, and fraud detection.
- E-commerce: Demand forecasts and recommender systems.
- Production: Predictive maintenance and process optimisation.
Using Data Analytics to Navigate
Data Analytics: Definition and Application
Data analytics is centred on analysing and interpreting past data to spot patterns, assess the results of choices or occurrences, and gauge how well a particular instrument or situation works. Comparatively speaking, it is a larger discipline that includes a variety of methods for deriving significant insights from structured datasets.
Fundamentals of Information Analytics
Analytics that are descriptive:
Summarising and analysing historical data to offer insights into past events is the goal of descriptive analytics. More sophisticated analytics are made possible by this fundamental step.
Diagnostic Analytics:
By examining past data, diagnostic analytics seeks to determine why specific events took place. Understanding the underlying reasons for observed outcomes entails spotting patterns and correlations.
Predictive Analytics:
Although it is an important part of Data Science, predictive analytics is also essential to Data Analytics. Based on past data, predictive models project future trends and results, assisting organisations in anticipating and becoming ready for what lies ahead.
Prescriptive Analytics:
Going beyond forecasting, prescriptive analytics makes recommendations for how best to achieve desired results. This entails making recommendations for actions or decisions in light of data-driven insights.
Various Industry Examples of Data Analytics
Data analytics is widely used in many different industries and helps with well-informed decision-making:
- Retail: Examining consumer purchasing trends to make more focused advertising efforts.
- Education: Assessing data on student performance to improve instruction.
- Sports: Using player data analysis to make tactical choices and boost output.
- Government: Allocating resources and providing public services more efficiently with data.
Read more:- Digitization
Key Differences Between Data Science and Data Analytics
Focus and Objectives
Data Science:
Focus: Predictive modelling and finding hidden patterns are the main areas of focus.
Objectives: The goals are to use cutting-edge statistical and machine learning approaches to extract insights, generate predictions, and automate decision-making processes.
Data Analytics:
Focus: Mostly on using historical data interpretation to guide decision-making.
Objectives: The goals are to comprehend historical patterns, spot connections, and offer practical advice for enhancing ongoing operations.
Skill Sets and Expertise Required
Data Science:
Skills: Expertise in machine learning techniques, sophisticated statistical understanding, and proficiency in programming languages (such as Python and R).
Expertise: Needs in-depth knowledge of mathematical modelling, intricate algorithms, and domain-specific information.
Data Analytics:
Skills: Mastery of statistical analysis, data visualisation, and technologies such as Excel, SQL, and BI platforms.
Expertise: Places a significant emphasis on interpreting data, applying statistical methods, and effectively communicating conclusions.
Tools and Technologies Used
Data Science:
Tools: TensorFlow, scikit-learn, Jupyter Notebooks, and specialised machine learning libraries are examples of tools.
Technologies: entails processing huge datasets using big data technologies (such as Hadoop and Spark).
Data Analytics:
Tools: Tableau, Power BI, Excel, and additional business intelligence instruments.
Technologies: To interpret and present insights, this field focuses on relational databases and data visualisation tools.
Time Horizon of Projects
Data Science:
Time Horizon: Consists of longer-term initiatives centred on creating and implementing prediction models.
Projects: Frequently use intricate machine learning models that require constant upkeep and modification.
Data Analytics:
Time Horizon: Projects with a shorter duration typically concentrate on analysing and presenting previous data.
Projects: Involve diagnostic and descriptive analytics to assist with urgent decision-making.
Decision-Making Processes
Data Science:
Decision Making: Using predictive models to automate decision-making procedures.
Impact: Has a long-term effect on strategic decisions.
Data Analytics:
Decision Making: Educates decision-makers by drawing on past patterns and trends.
Impact: Affects operational and tactical choices to enhance ongoing procedures.
Exploring Synergies Between Data Science and Data Analytics
Interconnectedness in the Data Science Lifecycle
Data Collection and Preprocessing:
- Contribution of Data Analytics: Data analytics can help ensure a strong basis for predictive modelling in data science by helping to understand the quality and structure of historical data.
Analysing exploratory data (EDA):
- Contribution of Data Analytics: By distilling and illustrating historical trends, descriptive analytics can contextualise exploratory data analysis (EDA) and assist data scientists in formulating hypotheses and narrowing their research focus.
Forecasting using Modelling:
- Contribution of Data Analytics: prediction analytics, a subset of data analytics, offers insights from past data that can direct the creation of prediction models that are more precise and efficient.
Using Analytics to Make Well-Informed Data Science Decisions
Analytics for diagnosis:
- Data Science Integration: By providing valuable insights into model performance problems, diagnostic analytics can help data scientists improve their models.
Analytics that prescribe:
- Data Science Integration: By offering useful insights from predictive modelling, prescriptive analytics recommendations can improve decision-making.
Increasing Cooperation to Boost Both Disciplines' Effectiveness
Interaction & Cooperation:
- Cross-disciplinary Cooperation: Encouraging efficient communication between data scientists and data analysts improves the data lifecycle as a whole and makes sure that insights from both fields are used to inform thorough decision-making.
Ongoing Education and Adjustment:
- Feedback Loop: For data science initiatives, data analytics offers useful feedback loops that facilitate ongoing model adaption and improvement depending on actual performance.
In summary
The differences between data science and data analytics become crucial in the complex web of data-driven decision-making, yet their potential for cooperation creates a dynamic coalition. We have discovered the distinctive qualities of every subject and emphasised their connections through our investigation, underscoring the need for a well-balanced integration.
Becoming an expert in one area is not the end of the journey in this age of unparalleled data availability. It is recommended that professionals adopt a multidisciplinary approach and acquire competencies in both data science and data analytics. This practice not only expands the capacities of individuals but also strengthens the ability of organisations as a whole to obtain valuable insights.
Anshul Goyal
Group BDM at B M Infotrade | 11+ years Experience | Business Consultancy | Providing solutions in Cyber Security, Data Analytics, Cloud Computing, Digitization, Data and AI | IT Sales Leader