What is data analytics? Techniques, Methods, and Key Differences
Data analytics transforms raw data into actionable insights, enabling businesses to make data-driven decisions and optimize operations.
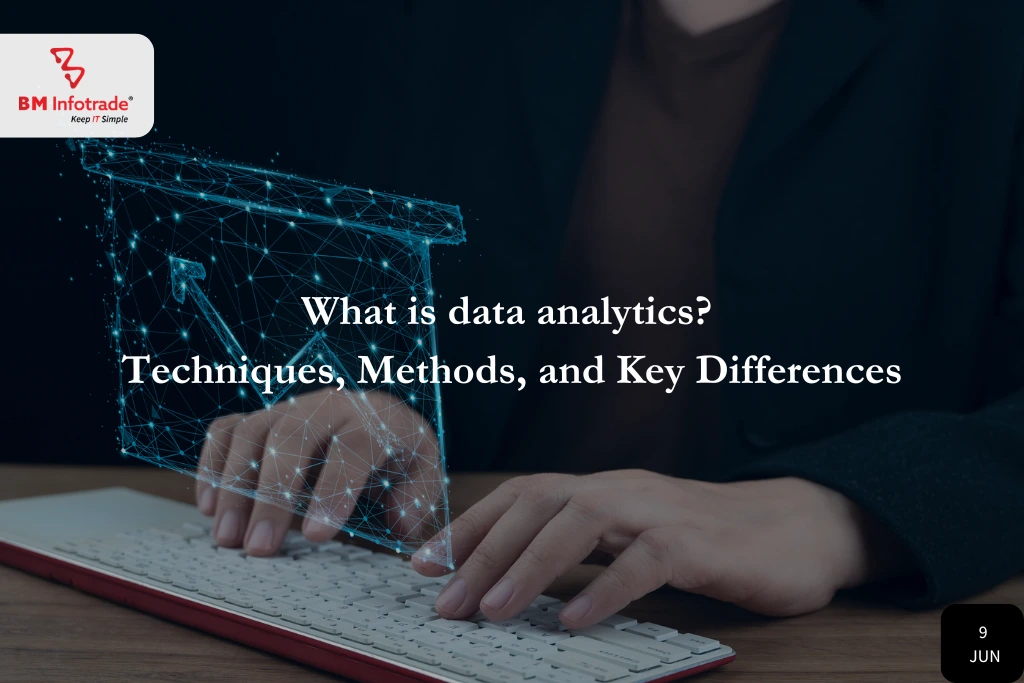
What is data analytics? Techniques, Methods, and Key Differences
Table of Contents
How Data Analytics Transforms Raw Data into Strategic Decisions?
The majority of organizations continuously collect massive volumes of data, yet this data in its unprocessed state doesn't directly show anything. One tool that helps in this is records analytics. Data analytics means the process of analyzing raw data to provide significant useful insights that can be utilized to guide and influence effective company choices.
A statistics analyst will take raw data, organize it, and then analyze it, transforming it from a collection of illogical numbers into a coherent body of knowledge. After analyzing the data, the data analyst will then present their conclusions in the form of advice or suggestions regarding what the company's next steps should be.
Data analytics can be thought of as a type of enterprise intelligence that is employed to address particular issues and difficult circumstances within a company. It all comes down to identifying patterns in a dataset that could reveal information about a specific area of the business—for example, how satisfied customers behave or how staff members interact with a particular piece of equipment.
Data analytics enables you to experience the future and predict future trends and behaviors; rather than basing your strategies and decisions on conjecture, you are making educated choices based on what the data is telling you.
Read More:- What is Cloud Computing? Its types? And how it helps companies?
Difference between Data Analytics and Data Science
Algorithms and machine learning are used by data scientists to improve the ways in which information supports business objectives. Data analysts collect, save, and retain information while also examining results.
The main distinction between statistical analytics and information technology is scope. Despite the fact that the two roles involve the same records units, a facts scientist's responsibilities are much more varied than a records analyst's. For that reason, records scientists frequently start out as facts analysts.
The questions the business should or could be asking, however, are taken into consideration by a facts scientist. They design novel information modeling techniques, create algorithms, create predictive models, and conduct specialized analyses. As an illustration, they might create a tool to use a dataset and automate specific actions based on the data, and then continuously track and examine it to make improvements and optimize it in any way that makes sense as new patterns and trends emerge.
Briefly stated, data scientists build systems to automate and optimize the overall operation of the commercial enterprise, while statistics analysts address and answer specific questions about facts on a regular basis. This allows them to reveal insights that other stakeholders can act upon.
The skills and abilities needed for each role represent another key point of differentiation. Software like Excel and, occasionally, programming languages like SQL, R, SAS, and Python are typically expected to be strong suit for data analysts. In order to perform database control, reporting, statistical analysis, and record mining, analysts need to feel comfortable using these tools and languages.
But data scientists are likely to be expected to be experts in Hadoop, Java, Python, machine learning, and object-oriented programming, along with software development, data mining, and information analysis.
What are methods of data analytics?
Descriptive analytics
The type of analysis known as descriptive analytics is straight forward and focuses on the surface level of past events. Data aggregation and data mining are the two main methods employed in descriptive analytics, so the data analyst first collects the data and presents it in a summarized format (that is, the aggregation part), and then "mines" the data to find patterns.
The information is then presented so that a large audience can understand it easily (not just data experts). The "what" must be determined and described at this point; cause-and-effect relationships are not attempted to be established or the historical data to be explained by descriptive analytics.
Diagnostic analytics
In contrast to diagnostic analytics, which focuses on the "why," descriptive analytics examines the "what." Data anomalies—i.e., anything that cannot be explained by the data in front of them—will be sought after first by data analysts when conducting diagnostic analytics. Examples include the data analyst will need to look into the reason if it appears that there was a sudden decline in sales for the month of March.
In order to accomplish this, they will start what is referred to as the discovery phase and look for any additional data sources that could provide them with additional information regarding the causes of the anomalies. Finally, the data analyst will try to identify causal relationships by, for instance, looking at any events that might be associated or correspond with the decline in sales.
Predictive analytics
The approach of predictive analytics focuses on the "what will" —to find future trends by combining descriptive, exploratory, diagnostic, machine learning, and artificial intelligence (AI) techniques. Using historical data, statistical modelling, data mining methods, and machine learning, this branch of advanced analytics aids firms in improving operational procedures and gaining an advantage over rivals.
Employing deep learning and machine learning techniques like decision trees, neural networks, and logistic and linear regression models, businesses employ predictive analytics to detect risks and opportunities. With the aid of sophisticated predictive analytics tools and models, organizations can accurately predict trends and behaviors in milliseconds, days, or even years in the future, improving their operating procedures and point of differentiation from the competition.
Prescriptive analytics
Prescriptive analytics, which builds on predictive analytics, focuses on the "how," and provides recommendations for the decisions and actions that should be taken. Prescriptive analytics then gives you advice on how to make the most of the results that were expected. When doing prescriptive evaluation, information analysts will consider possible results and potential organizational responses.
One of the more complex types of analysis is prescriptive analytics, which may involve using computational modeling techniques, device learning, and algorithmic operation. However, the employer's system for making hiring decisions as well as the bottom line may be significantly impacted by the effective use of prescriptive analytics.
Read More:- How Business Continuity Plan affect our lives?
Conclusion
The availability of Big Data, the low cost of commodity technology, and new information management and analytical software have made this the most advantageous time in the history of data analysis. As a result of the convergence of these tendencies, we now have the resources available to analyze huge data sets fast and economically for the first time in history. These skills don't just exist in theory or as a formality. They represent a genuine development and an obvious opportunity to make major gains in productivity, revenue, profitability, and efficiency.
The Big Data era has come, and if industry experts in business and technology work together to fulfill the promise, then these are truly revolutionary times.
Anshul Goyal
Group BDM at B M Infotrade | 11+ years Experience | Business Consultancy | Providing solutions in Cyber Security, Data Analytics, Cloud Computing, Digitization, Data and AI | IT Sales Leader