Cloud Analytics: the deployment of scalable cloud computing
Discover the role of cloud analytics in deploying scalable cloud computing solutions. Explore how businesses leverage data analysis for improved decision-making and efficiency.
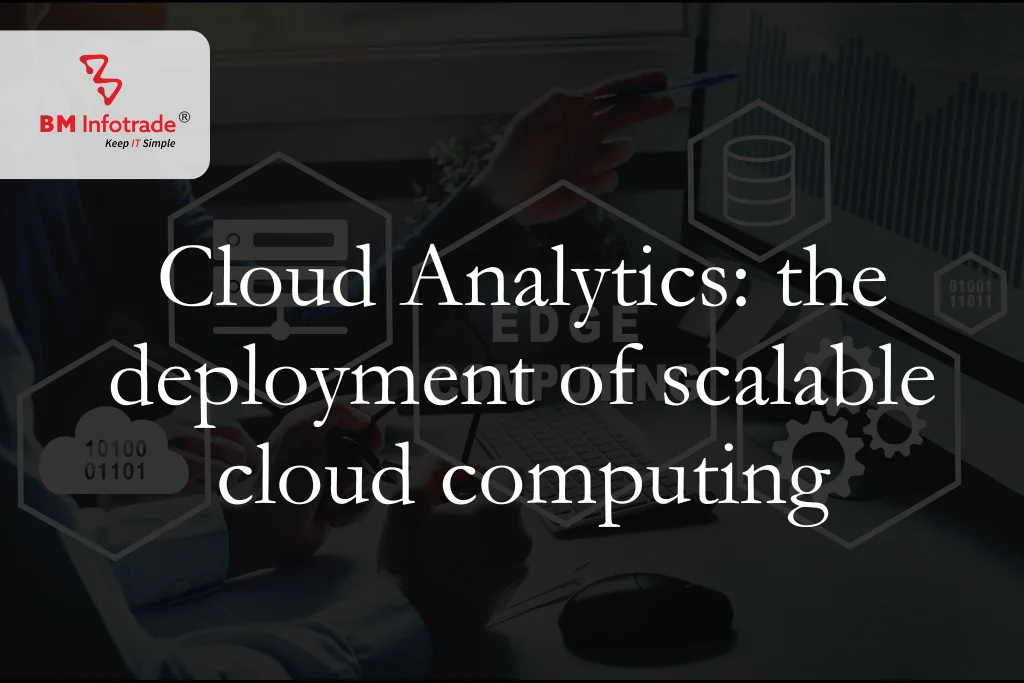
Cloud Analytics: the deployment of scalable cloud computing
Cloud analytics is the practice of applying analytic algorithms to data stored in a private or public cloud and then delivering the desired outcome. To find patterns in data and derive fresh insights, cloud analytics entails the deployment of scalable cloud computing with robust analytical software. Data analysis is increasingly used by businesses to gain a competitive edge, advance scientific research, and improve people's lives in a variety of ways. Consequently, as the amount and value of data continue to rise, data analytics has grown in importance as a tool.
Artificial intelligence (AI), machine learning (ML), and deep learning are frequently linked to cloud analytics (DL). Additionally, it is frequently used in commercial applications, including business intelligence, security, the Internet of Things (IoT), genomics research, and work in the oil and gas industry. In fact, data analytics can boost organizational performance and create new value in any sector.
Organizations of all sizes can quickly make data-driven decisions to improve the efficiency of their products and services by utilizing AI and other analytics techniques. The cloud is a crucial platform that enables rapid idea experimentation through proofs of concept (POCs) and offers a robust software ecosystem for developing AI applications and training DL models.
In order to analyze data, this refers to the use of cloud-based tools and services. Both data that is streamed to the cloud for analysis and data that is stored there can fall under this category. It may contain information gathered from sensors, mobile devices, and on-premises applications.
Using analytical algorithms, cloud analytics analyzes data that is stored in the cloud. Cloud analytics brings together scalable cloud computing with potent analytics software in order to find patterns in data and derive new insights. Businesses have relied on cloud-based data analysis more and more over the past two years or so.
Table of Contents
How cloud analytics can promote scalability and growth
The idea of data analytics is not new. The phrase "big data" was first used in the late 1990s to refer to the sizeable data sets that are frequently found in a variety of sectors, including energy, financial, healthcare, spaceflight, and other scientific fields. Data analytics, or the process of analyzing and drawing conclusions from large data sets, has become easier thanks to the development of analytics programs like Apache Hadoop. Analytics workloads and technologies that were moved to the cloud are now referred to as cloud analytics. The capability, accessibility, and ease of performing complex data analysis on very large data sets have all increased significantly thanks to cloud analytics.
- The amount of data being collected globally is increasing at startling rates, and a large portion of it is being created and gathered in the cloud or at IOT endpoints.
- Because cloud services are delivered as automated services and don't require the deployment and upkeep of physical hardware, they are much simpler to deploy.
- A user can turn services on and off as needed thanks to the cloud business model. The cost of purchasing and managing capital infrastructure is removed, and the need for data center space is reduced, thanks to this consumption-based pricing model. Users can use the cloud to deploy the appropriate amount of IT resources based on the issue at hand.
- With the help of the cloud, users can allocate the appropriate number of IT resources to the current issue. Users can easily apply compute and storage and scale them as needed thanks to dynamic resource sizing. For all of their data analysis projects, users are not required to purchase a fixed capacity of physical IT equipment.
- Users who want to use the cloud to test a new analytics project as a POC before making investments on-premises should build a hybrid analytics solution.
Organizations can use cloud analytics to do the following:
- Test genomic data to better understand genetic disease and how to provide treatments
- Recognize patterns in speech, images, and videos to enhance customer service and satisfaction.
Read more: Basics of Network Technologies: Benefits of Networks
Cloud Analytics Types
For instance, organizations that must react quickly to real-time events can use on-demand analytics services for real-time decision-making in a closed-loop system. Mission-critical industries and business processes typically use on-demand analytics services. These organizations must make decisions quickly because their environment is changing at an incredibly fast rate.
Four categories of cloud analytics:
- Analytics in the public cloud: The public cloud is the best option for businesses that need to act quickly and have a limited budget. It can handle large data sets, is economical, and makes use of cutting-edge technology.
- Private cloud analytics: This gives your company a more streamlined and secure way to analyze data.
- Hybrid cloud: Data may be kept on systems that are both on-premises and in the cloud. These data can be examined using cloud-based analysis tools. A mix of public and private infrastructure is used to deliver services in an on-demand cloud analytics service. Information is secured and secure connectivity with end users is made possible by using private hardware and software.
- Edge cloud: In the edge cloud, data is examined as it is being received rather than at a later time. It might consist of a sensor, server system, handheld device, or other mobile devices.
Read more: What does software testing do, and what significance does it hold
Conclusion-
Today, data is the key to successful business operations. Data is being used by every organization to make business decisions. Azure Synapse Analytics provides a limitless analytics service that combines data ingestion, enterprise data warehousing, and big data analytics using the enormous increase in data being generated and collected by organizations from a variety of data sources (whether it is structured, semi-structured, or unstructured data).
Anshul Goyal
Group BDM at B M Infotrade | 11+ years Experience | Business Consultancy | Providing solutions in Cyber Security, Data Analytics, Cloud Computing, Digitization, Data and AI | IT Sales Leader