Types of Data Analytics: A Comprehensive Guide
Discover the various types of data analytics, including descriptive, diagnostic, predictive, and prescriptive analytics. Learn how they contribute to informed decision-making and business success.
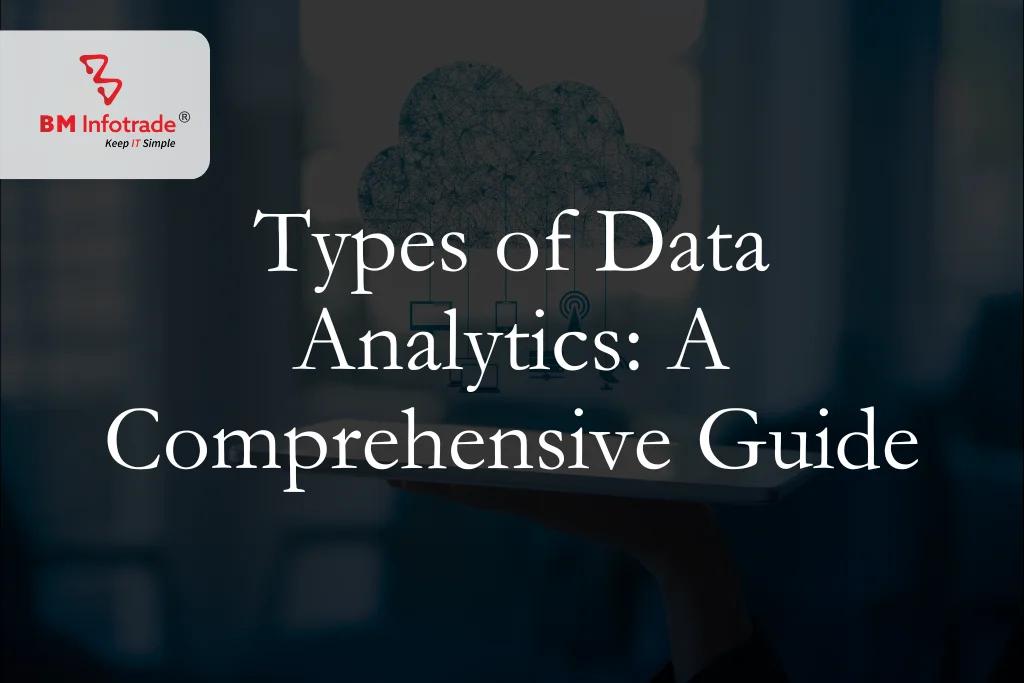
Types of Data Analytics: A Comprehensive Guide
Types of data analytics are crucial across industries in the ever-changing world of data-driven decision-making. Data analytics helps companies increase market share, improve strategic planning, and obtain insights. This thorough book covers data analytics' definitions, applications, and importance in today's data-centric environment.
Data analytics uses methods and tools to analyse, evaluate, and act on raw data. Datasets are systematically examined to find patterns, correlations, and trends. Organizations may enhance processes, make choices, and find opportunities by turning raw data into insights.
Data analytics is important in healthcare, finance, e-commerce, marketing, and more. Data analytics boosts efficiency, customer satisfaction, and creativity in businesses and institutions. In today's competitive market, data power is crucial.
Data analytics is complex, and this blog will explore its forms and uses. Start our research with "Descriptive Analytics," a core part of data analytics that delivers insights into historical data, providing a glimpse of the past. Let's explore how descriptive analytics helps reveal data stories.
Table of Contents
Types of Data Analytics
Descriptive Analytics
Data analysis begins with descriptive analytics, which provides a retrospective view of historical data to reveal prior events and trends. This sort of analytics summarises and interprets data to define the "what" of a situation, helping organisations understand their performance and make informed decisions based on historical context.
Diagnostic Analytics
Let's move on to "Diagnostic Analytics," a vital step that goes beyond the 'what' to discover the 'why' behind specific outcomes. Diagnostic analytics examines prior occurrences to find patterns, connections, and causality. By investigating trends and events, organisations can better understand their operations and make improvements.
Diagnostic analytics incorporates probing questions and root cause analysis, like data detective work. This section defines, explains, and applies diagnostic analytics, showing how it's an essential tool in data analytics.
Predictive Analytics
We'll next investigate "Predictive Analytics." Predictive analytics uses historical data and statistical algorithms to anticipate future results, unlike descriptive and diagnostic analytics. Advanced modelling helps organisations predict trends, detect hazards, and make proactive decisions in a fast-changing environment.
Read more: What is the work of a Data Analyst? Assemble data and embrace excellence.
Techniques and methods
Statistical Analysis
Data analytics relies on statistical analysis to collect, analyse, interpret, present, and organise data. Statistics are used to find patterns, connections, and trends in datasets. Whether descriptive statistics summarise data or inferential statistics predict it, statistical analysis helps get insights from complex datasets.
Machine Learning
Welcome to "Predictive Analytics," a sophisticated data analytics subset that uses machine learning. Predictive analytics uses algorithms and statistical models to predict future outcomes using historical data. Organizations can make proactive decisions and strategic plans by training models on prior patterns.
Data Mining
Data mining uses machine learning, statistics, and database systems to find patterns and information in massive datasets. It uncovers data patterns and linkages alongside predictive analytics. From clustering to association rule mining, data mining techniques help decision-makers discover insights.
Text Analytics
Text analytics, often known as text mining or natural language processing, extracts meaning from unstructured text data. It helps extract insights from papers, social media, emails, and other textual sources. In this section, we'll learn how text analytics helps understand customer sentiment, extract useful information, and improve decision-making.
Read more: Database: Collecting all the data in one place.
Industry Data Analytics Users
Health
Data analytics transforms healthcare delivery, patient outcomes, and operations. Diagnostic analytics explains health trends, while descriptive analytics summarises patient data. Disease outbreaks and patient readmissions are predicted using predictive analytics.
Finance
Data analytics, especially predictive analytics, transforms finance. Finance companies use predictive models for credit scoring, fraud detection, and investment portfolio optimisation. Diagnostic analytics looks for the reasons of financial irregularities, whereas descriptive analytics summarises past financial data.
E-commerce
Data analytics drives personalised customer experiences in e-commerce. Predictive analytics helps e-commerce platforms recommend products based on user preferences. Diagnostic analytics identifies purchase decision factors, whereas descriptive analytics summarises customer behaviour.
Marketing
Data analytics drives client segmentation and customised campaigns in marketing. Predictive analytics predicts customer behaviour, while descriptive analytics analyses historical marketing performance. Diagnostic analytics helps marketers evaluate marketing channels.
Issues and Considerations
Security and Privacy of Data
Data privacy and security are the biggest issues as data analytics become more common. As organisations collect and analyse massive volumes of sensitive data, data security is crucial. This section will discuss data privacy and security in data analytics, including changing rules and ethical issues.
Data Analytics Ethics
Responsible data analytics requires ethics. Organizations using data to make choices must consider the ethical consequences. Data analytics ethics include algorithm bias, transparency in decision-making, and responsible data use to avoid unintended consequences.
Training requirements and skill gap
Rapid data analytics technology advancement generates a skills gap to maximise their potential. This section will cover the data analytics skill gap and the need for statistics, machine learning, and data visualisation experts. It will also examine how individuals and organisations may keep current in data analytics training.
Future Data Analytics Trends
AI Integration
The field will be transformed by AI-data analytics integration. AI algorithms improve pattern recognition, predictive modelling, and decision-making. This section discusses how AI and data analytics are transforming the future and enabling more intelligent and automated insights.
Edge Analytics
The demand for real-time analytics has led to edge analytics as data grows exponentially. Data analysis at the source reduces latency and speeds decision-making. We'll discuss edge analytics, its uses, and how it meets industries' immediate insight needs.
Automation Rise
Automation is growing in data analytics, from data preparation to model deployment. In this section, we'll examine the benefits, drawbacks, and potential effects of data analytics workflow automation on data professionals. Automation will streamline processes, helping organisations gain insights faster.
Conclusion
We explored data analytics' many types and uses in this detailed guide. Our analytical approaches range from Descriptive Analytics, which provides insights into historical data, to Diagnostic Analytics, which investigates deeper, Predictive Analytics, which predicts the future, and predictive Analytics, which makes actionable recommendations.
Growing relevance of data analytics. Data-driven insights are helping companies across industries make better decisions, boost efficiency, and compete. We discover through statistical analysis, machine learning, data mining, and text analytics that data analytics' power rests in its ability to turn raw data into actionable intelligence.
Continuous learning and adaptation are crucial as data analytics evolves. Organizations and individuals must adapt to technological changes by addressing data privacy and ethics issues and bridging the skill gap through training.
In conclusion, this guide covered data analytics' issues, considerations, and future developments. We've examined how data visualisation improves interpretability and looked ahead to artificial intelligence, edge analytics, and process automation.
Anshul Goyal
Group BDM at B M Infotrade | 11+ years Experience | Business Consultancy | Providing solutions in Cyber Security, Data Analytics, Cloud Computing, Digitization, Data and AI | IT Sales Leader