Breaking Down the Generative AI Vs Traditional AI
Dive into the contrasts between generative AI and traditional AI. Learn how each approach functions and their unique applications in today’s tech landscape.
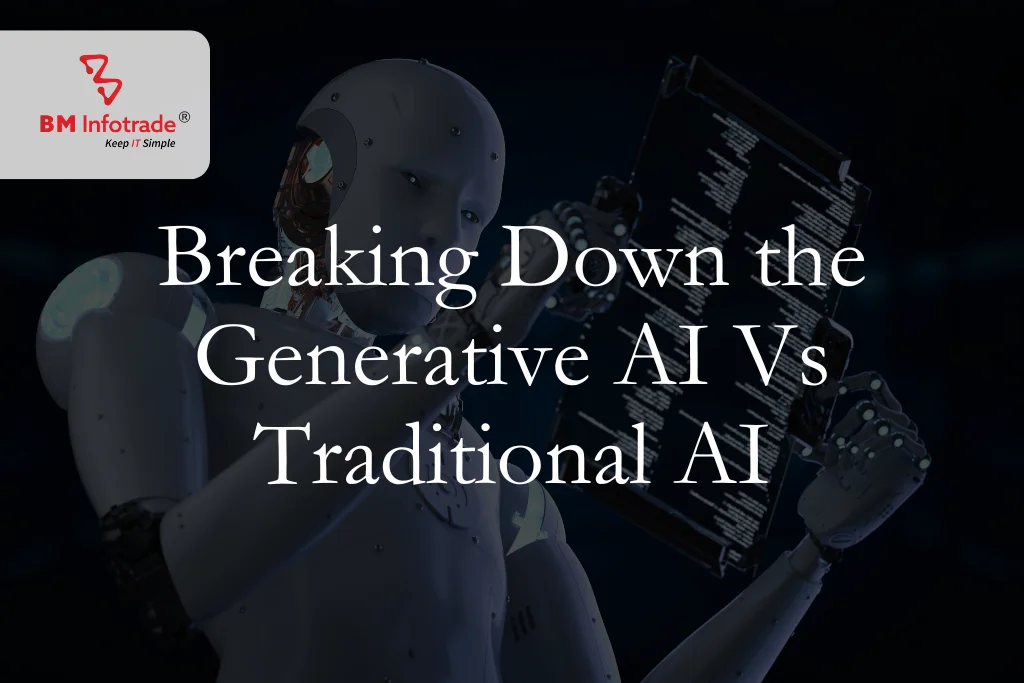
Breaking Down the Generative AI Vs Traditional AI
Here we talk about generative AI and Traditional AI; these are hot topics in recent times, and we will go in deep and understand the AI journey. Robot perception, learning, and decision-making are being transformed by disruptive technologies like AI. Traditional and generative AI must be distinguished while researching AI.
AI includes a wide range of technologies that mimic human intellect. From rule-based systems to neural network designs, AI has evolved greatly.
Early deterministic rule-based systems gave way to more advanced learning paradigms in AI. The development allows a thorough comparison between Generative AI and Traditional AI.
Traditional AI with rule-based systems and symbolic representations will be examined in this study. Generative AI, where data learning dominates, will change problem-solving.
Table of Contents
Traditional AI
Systems based on Rules
Conventional AI frequently uses rule-based systems, in which the behaviour of the system is determined by explicit programming. These systems handle issues and make decisions based on a preset set of rules, usually expressed as IF-THEN expressions.
The basis of rule-based systems is the logic of the system being built around human skill and knowledge. Engineers work very hard to create rules that span a range of situations and try to mimic human thought and judgement.
Symbolic AI
Another pillar of traditional AI is symbolic AI, which is concerned with modelling knowledge and emulating intelligent behaviour through the manipulation of symbols. One well-known use of symbolic AI is in expert systems, which imitate human competence in certain fields by using symbolic representations of knowledge.
Formal logic, ontologies, or semantic networks are used in symbolic artificial intelligence (AI) to organise knowledge and allow the system to reason, infer, and draw conclusions from the data at hand.
Conventional AI's Limitations
Even with these improvements, Traditional AI still has drawbacks, mainly because it depends too much on explicit programming and pre-established rules. When placed in unfamiliar circumstances or contexts that deviate from their preprogrammed norms, these systems find it difficult to adjust.
Traditional AI is too inflexible to learn from data on its own; instead, it needs regular human involvement to update rules and take new information into account.
When Traditional AI is contrasted with Generative AI, it is clear that the former functions in an organised manner under the control of preset guidelines and symbolic representations, whereas the latter takes a more dynamic approach, utilising data-driven learning mechanisms to produce original solutions and adjust to changing problems.
Read More: Types of Data Analytics: A Comprehensive Guide
Generative AI
Definition and Principles
A paradigm change is represented by generative AI, which places more emphasis on data-driven learning than explicit programming. Fundamentally, it makes use of neural networks—a class of algorithms inspired by the structure of the human brain—to produce results that are meaningful and discover patterns on its own.
The basic idea behind generative AI is to feed a model enormous quantities of data so that it may learn and extract features. This will enable the model to produce new content or make predictions without needing to be explicitly programmed for a specific job.
Types of Generative AI
Generative Adversarial Networks, or GANs: A GAN has a generator and discriminator neural network. They compete. The discriminator evaluates generator-generated data. Antagonistic training produces realistic content.
VAEs (Variational encoders): Probabilistic Variational encoders (VAEs) encode and decode data. Virtual Autoencoders (VAEs) learn the distribution of input data and produce new, similar data points for probabilistic and expressive generative applications.
Generative AI Applications
Generation of Images and films: Generative AI creates realistic visuals and videos. Deepfake technology and art production create ethical concerns.
Natural Language Processing: Generative AI understands and reproduces human-like speech patterns in text generation, language translation, and conversation systems.
Advantages of Generative AI
Creativity & Innovation: Innovative and creative outcomes that surpass preconceived notions are produced by generative AI, which thrives in creative domains.
Adaptability and Self-Improvement: Generative AI is more adaptable than Traditional AI, learning new abilities and improving old ones with new data. This versatile creature can adjust swiftly to shifting surroundings.
Read More: The Future of Data Analytics: Career Opportunities in 2024
Differences between Generative AI and Traditional AI
Approach to Problem-Solving
Explicit Rules vs. Data-Based Learning: Conventional AI follows human programmers' guidelines. These recommendations arrange problem-solving by defining system behaviour in distinct contexts. Instead, generative AI uses massive datasets to learn from and automatically detect patterns and correlations to solve problems without rules.
Adaptability and Flexibility
Predefined Structures vs. Continuous Learning: Conventional AI systems find it difficult to adjust to novel or unexpected situations because they are limited by predefined rules. With its data-driven methodology, generative AI exhibits greater flexibility. By continually learning from incoming data, it may modify its behaviour and get better over time, increasing its resilience in changing situations.
Originality and Imagination
Rule-Bound vs. Generative Exploration: Explicit rules and structured programming are the parameters in which traditional AI functions. Although it works well for jobs with rules, it frequently lacks the ability to explore creatively. On the other hand, generative AI is very useful in creative industries like art, design, and content creation because of its amazing capacity to produce original and innovative products.
Use Cases and Examples
Particular Uses for Conventional AI: Conventional AI finds use in activities that are well-defined and governed by rules, such automated decision-making in manufacturing, structured data processing, and expert systems for diagnosis.
Innovative Uses Made Possible by Generative AI: Adaptive systems that learn and change in response to user inputs, realistic picture and video production, and natural language processing with creative text generation are just a few of the creative uses made possible by generative AI.
When comparing Generative AI with Traditional AI, it becomes clear that the former's rule-centric, preset structure and the latter's data-driven, autonomous learning approach are very different from one another. These differences have significant effects on creativity, flexibility, and problem-solving skills, as well as the breadth of applications that each paradigm can successfully handle.
Challenges and Considerations
Ethical Concerns
Bias in Data and Algorithms: Training data biases can affect both traditional AI systems and generative AI systems. When these prejudices are reinforced and result in discriminatory effects, ethical issues become apparent. It becomes imperative to address prejudice in AI systems in order to guarantee equity and justice in decision-making procedures.
Accountability in Decision-Making: Determining and assigning responsibility for the decisions made by AI systems, particularly Generative AI, presents a substantial issue as these systems get more independent. It becomes essential to set up responsible and transparent frameworks in order to reduce any possible dangers related to decisions or material created by AI.
Computational Requirements
Resource-Intensive Nature of Generative Models: Generic artificial intelligence, especially models such as GANs, frequently requires significant computer power. Strong hardware is needed for training and implementing these models, which raises questions regarding accessibility and environmental effect. One of the main challenges is juggling the computing needs of generative AI with environmental considerations.
Challenges with Scalability: Developing Generative AI models for practical use presents scalability and efficiency issues. Widespread use of the technology depends on its continued practicality and scalability as dataset sizes and model complexity rise.
It's crucial to face the difficulties and moral dilemmas that come with both traditional and generative AI when navigating the AI environment. To fully use new technologies and reduce related hazards, it is critical to strike a balance between innovation, ethical use, and practical factors. To guarantee AI's beneficial effects on society, the field is still developing and requires vigilance and responsibility.
Future Outlook
Possible Developments in Artificial Intelligence
Improvements to Model Capabilities: Research and development should yield increasingly powerful and flexible Generative AI models. As computer vision, natural language processing, and creativity progress, it may become harder to discern between AI and human-created content.
Multidisciplinary Integration: Combining generative AI with VR and AR can lead to creative applications. This interdisciplinary convergence may change our interactions with AI-generated media through immersive and interactive experiences.
Combining Generative and Conventional AI
Hybrid Approaches: By fusing the best aspects of Generative AI with Traditional AI, it may be possible to create hybrid systems that combine the adaptability and creativity of generative models with organised rule-based reasoning. This integration might lead to more resilient AI systems that perform better across a larger range of jobs.
Complementary Solutions: Future AI applications may smoothly combine Traditional AI for rule-bound work with Generative AI for creative, data-driven jobs, acknowledging the advantages and disadvantages of each paradigm. AI systems that offer comprehensive and efficient answers may be the product of this peaceful cooperation.
Effects on Different Industries and the Community
Transformation in Creative Industries: The creative sectors, including art, design, and entertainment, are predicted to undergo a significant transformation due to the potential of generative AI to stimulate creativity. We foresee the advent of fresh approaches to digital art, creative designs, and interactive narratives powered by AI-generated material.
Improved Business Decision-Making: Traditional and generative AI might revolutionise commercial decision-making. AI technologies are projected to streamline operations and promote innovation across industries, from data-driven insights to adaptive strategies.
Future AI trends include continual advancement, interdisciplinary cooperation, and revolutionary impact on industries and communities. This shifting landscape requires a forward-thinking approach, ethical considerations, and a commitment to optimising AI's human benefits.
Conclusion
Finally, the divide between Generative AI and Traditional AI shows how artificial intelligence has evolved. Traditional AI, based on rule-based systems and symbolic representations, excels at prescribed tasks but struggles with adaptation and creativity. Generative AI, with its data-driven learning and autonomous capabilities, promotes innovation and creativity but must consider ethical and computational issues.
With Generative AI capabilities improving and Traditional AI being thoughtfully integrated, AI may see synergies between both paradigms. We must balance computational needs, ethical concerns, and responsibility in this setting. Generative AI and Traditional AI working together might lead to a future where intelligent systems help solve complicated issues, innovate, and improve decision-making across sectors.
Anshul Goyal
Group BDM at B M Infotrade | 11+ years Experience | Business Consultancy | Providing solutions in Cyber Security, Data Analytics, Cloud Computing, Digitization, Data and AI | IT Sales Leader